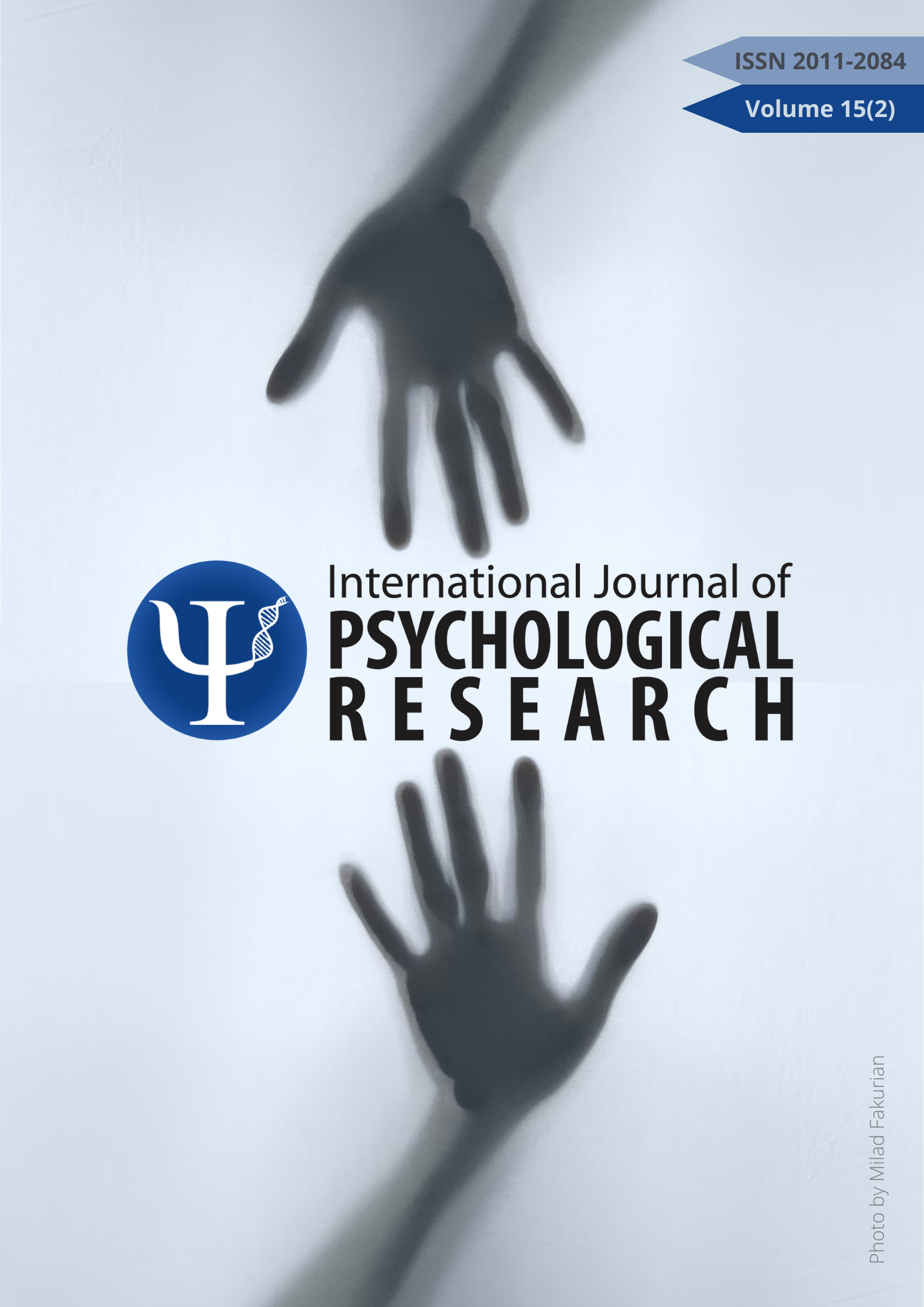
To give up copyright, the authors allow that, International Journal of Psychological Research, distribute the work more broadly, check for the reuse by others and take care of the necessary procedures for the registration and administration of copyright; at the same time, our editorial board represents the interests of the author and allows authors to re-use his work in various forms. In response to the above, authors transfer copyright to the journal, International Journal of Psychological Research. This transfer does not imply other rights which are not those of authorship (for example those that concern about patents). Likewise, preserves the authors rights to use the work integral or partially in lectures, books and courses, as well as make copies for educational purposes. Finally, the authors may use freely the tables and figures in its future work, wherever make explicit reference to the previous publication in International Journal of Psychological Research. The assignment of copyright includes both virtual rights and forms of the article to allow the editorial to disseminate the work in the manner which it deems appropriate.
The editorial board reserves the right of amendments deemed necessary in the application of the rules of publication.
Abstract
The primary purpose of the present study was to inform and illustrate, using examples, the use of Diagnostic Classification Models (DCMs) for the assessment of skills and competencies in cognition and academic achievement. A secondary purpose was to compare and contrast traditional and contemporary psychometrics for the measurement of skills and competencies. DCMs are described along the lines of other psychometric models within the Confirmatory Factor Analysis tradition, such as the bifactor model and the known mixture models that are utilized to classify individuals into subgroups. The inclusion of interaction terms and constraints along with its confirmatory nature enables DCMs to accurately assess the possession of skills and competencies. The above is illustrated using an empirical dataset from Saudi Arabia (n = 2,642), in which language skills are evaluated on how they conform to known levels of competency, based on the CEFR (Council of Europe, 2001) using the English Proficiency Test (EPT).
Keywords:
References
Alexander, G. E., Satalich, T. A., Shankle, W. R., & Batchelder, W. H. (2016). A cognitive psychometric model for the psychodiagnostic assessment of memory-related deficits. Psychological assessment, 28 (3), 279. https://doi.org/10.1037/pas0000163
Asparouhov, T., & Muthén, B. (2009). Exploratory structural equation modeling. Structural Equation Modeling, 16, 397–438. https://doi.org/10.1080/10705510903008204
Bonifay, W., & Cai, L. (2017). On the complexity of item response theory models. Multivariate behavioral research, 52 (4), 465–484. https://doi.org/10.1080/00273171.2017.1309262
Bower, J., Runnels, J., Rutson-Griffiths, A., Schmidt, R., Cook, G., Lehde, L., & Kodate, A. (2017). Aligning a Japanese university’s English language curriculum and lesson plans to the CEFR-J. In F. O’Dwyer, M. Hunke, A. Imig, N. Nagai, N. Naganuma, & M. G. Schmidt (Eds.), Critical, Constructive Assessment of CEFR-informed Language Teaching in Japan and Beyond (pp. 176–225). Cambridge University Press.
Bozard, J. L. (2010). Invariance testing in diagnostic classification models (Doctoral dissertation). The University of Georgia. https://getd.libs.uga.edu/pdfs/bozard_jennifer_l_201005_ma.pdf
Bradshaw, L., Izsák, A., Templin, J., & Jacobson, E. (2014). Diagnosing teachers’ understandings of rational numbers: Building a multidimensional test within the diagnostic classification framework. Educational measurement: Issues and practice, 33 (1), 2–14. https://doi.org/10.1080/15305058.2015.1107076
Bradshaw, L. P., & Madison, M. J. (2016). Invariance properties for general diagnostic classification models. International Journal of Testing, 16 (2), 99–118. https://doi.org/10.1080/15305058.2015.1107076
Chen, Y., Liu, J., Xu, G., & Ying, Z. (2015). Statistical analysis of Q-matrix based diagnostic classification models. Journal of the American Statistical Association, 110 (510), 850–866. https://doi.org/10.1080/01621459.2014.934827
Council of Europe. (2001). Common European Framework of Reference for Languages: Learning, teaching, assessment. Cambridge University Press.
Davier, M. V. (2009). Some notes on the reinvention of latent structure models as diagnostic classification models. Measurement: Interdisciplinary Research and Perspectives, 7 (1), 67–74. https://doi.org/10.1080/15366360902799851
DiBello, L. V., Henson, R. A., & Stout, W. F. (2015). A family of generalized diagnostic classification models for multiple choice option-based scoring. Applied Psychological Measurement, 39 (1), 62–79. https://doi.org/10.1177%2F0146621614561315
Emons, W. H., Glas, C. A., Meijer, R. R., & Sijtsma, K. (2003). Person fit in order-restricted latent class models. Applied psychological measurement, 27 (6), 459–478. https://doi.org/10.1177%2F0146621603259270
Gierl, M. J., Alves, C., & Majeau, R. T. (2010). Using the attribute hierarchy method to make diagnostic inferences about examinees’ knowledge and skills in mathematics: An operational implementation of cognitive diagnostic assessment. International Journal of Testing, 10 (4), 318–341. https://doi.org/10.1080/15305058.2010.509554
Gorin, J. S., & Embretson, S. E. (2006). Item difficulty modeling of paragraph comprehension items. Applied Psychological Measurement, 30, 394–411. https://doi.org/10.1177/0146621606288554
Gorsuch, R. (1983). Factor analysis. Lawrence Erlbaum Associates.
Hansen, M., Cai, L., Monroe, S., & Li, Z. (2016). Limited information goodness-of-fit testing of diagnostic classification item response models. British Journal of Mathematical and Statistical Psychology, 69 (3), 225–252. https://doi.org/10.1111/bmsp.12074
Hasselgreen, A. (2013). Adapting the CEFR for the classroom assessment of young learners’ writing. The Canadian Modern Language Review, 69, 415–435. https://doi.org/10.3138/cmlr.1705.415
Henson, R., DiBello, L., & Stout, B. (2018). A Generalized Approach to Defining Item Discrimination for DCMs. Measurement: Interdisciplinary Research and Perspectives, 16 (1), 18–29. https://doi.org/10.1080/15366367.2018.1436855
Huang, H. Y. (2017). Multilevel cognitive diagnosis models for assessing changes in latent attributes. Journal of Educational Measurement, 54 (4), 440–480. https://doi.org/10.1111/jedm.12156
Jang, E. (2009). Cognitive diagnostic assessment of L2 reading comprehension ability: Validity arguments for Fusion Model application to LanguEdge assessment. Language Testing, 26, 31–73. https://doi.org/10.1177%2F0265532208097336
Jurich, D. P., & Bradshaw, L. P. (2014). An illustration of diagnostic classification modeling in student learning outcomes assessment. International Journal of Testing, 14 (1), 49–72. https://doi.org/10.1080/15305058.2013.835728
Kaya, Y., & Leite, W. L. (2017). Assessing change in latent skills across time with longitudinal cognitive
diagnosis modeling: An evaluation of model performance. Educational and psychological measurement, 77 (3), 369–388. https://doi.org/10.1177%2F0013164416659314
Köhn, H. F., & Chiu, C. Y. (2018). How to Build a Complete Q-Matrix for a Cognitively Diagnostic Test. Journal of Classification, 35 (2), 273–299. https://doi.org/10.1007/s00357-018-92550
Kunina-Habenicht, O., Rupp, A. A., & Wilhelm, O. (2009). A practical illustration of multidimensional diagnostic skills profiling: Comparing results from confirmatory factor analysis and diagnostic classification models. Studies in Educational Evaluation, 35 (2-3), 64–70. https://doi.org/10.1016/j.stueduc.2009.10.003
Kusseling, F., & Lonsdale, D. (2013). A corpus-based assessment of French CEFR lexical content. The Canadian Modern Language Review, 69, 436–461. https://doi.org/10.3138/cmlr.1726.436
Little, D. (2007). The common European framework of reference for languages: Perspectives on the making of supranational language education policy. The Modern Language Journal, 91, 645–655. https://doi.org/10.1111/j.1540-4781.2007.00627_2.x
Liu, R., Huggins-Manley, A. C., & Bradshaw, L. (2017). The impact of Q-matrix designs on diagnostic classification accuracy in the presence of attribute hierarchies. Educational and psychological measurement, 77 (2), 220–240. https://doi.org/10.1177%2F0013164416645636
Liu, R., Huggins-Manley, A. C., & Bulut, O. (2018). Retrofitting diagnostic classification models to responses from IRT-based assessment forms. Educational and psychological measurement, 78 (3), 357–383. https://doi.org/10.1177%2F0013164416685599
Madison, M. J., & Bradshaw, L. P. (2015). The effects of Q-matrix design on classification accuracy in the log-linear cognitive diagnosis model. Educational and Psychological Measurement, 75 (3), 491–511. https://doi.org/10.1177%2F0013164414539162
McGill, R. J., Styck, K. M., Palomares, R. S., & Hass, M. R. (2016). Critical issues in specific learning disability identification: What we need to know about the PSW model. Learning Disability Quarterly, 39 (3), 159–170. https://doi.org/10.1177%2F0731948715618504
Rupp, A. A., & Templin, J. L. (2008). Unique characteristics of diagnostic classification models: A comprehensive review of the current state-of-the-art. Measurement, 6 (4), 219–262. https://doi.org/10.1080/15366360802490866
Sessoms, J., & Henson, R. A. (2018). Applications of Diagnostic Classification Models: A Literature Review and Critical Commentary. Measurement: Interdisciplinary Research and Perspectives, 16 (1), 1–17. https://doi.org/10.1080/15366367.2018.1435104
Templin, J., & Bradshaw, L. (2013). Measuring the reliability of diagnostic classification model examinee estimates. Journal of Classification, 30 (2), 251–275. https://doi.org/10.1007/s00357-0139129-4
Templin, J., & Hoffman, L. (2013). Obtaining diagnostic classification model estimates using Mplus. Educational Measurement: Issues and Practice, 32 (2), 37–50. https://doi.org/10.1111/emip.12010
Tu, D., Gao, X., Wang, D., & Cai, Y. (2017). A new measurement of internet addiction using diagnostic classification models. Frontiers in psychology, 8, 1768. https://doi.org/10.3389%2Ffpsyg.2017.01768
Walker, G. M., Hickok, G., & Fridriksson, J. (2018). A cognitive psychometric model for assessment of picture naming abilities in aphasia. Psychological assessment, 30 (6), 809–826. https://doi.org/10.1037%2Fpas0000529
Wang, C. (2013). Mutual information item selection method in cognitive diagnostic computerized adaptive testing with short test length. Educational and Psychological Measurement, 73 (6), 1017–1035. https://doi.org/10.1177%2F0013164413498256
Xia, Y., & Zheng, Y. (2018). Asymptotically Normally Distributed Person Fit Indices for Detecting Spuriously High Scores on Difficult Items. Applied psychological measurement, 42 (5), 343–358. https://doi.org/10.1177%2F0146621617730391
Xie, Q. (2017). Diagnosing university students’ academic writing in English: Is cognitive diagnostic modeling the way forward? Educational Psychology, 37 (1), 26–47. https://doi.org/10.1080/01443410.2016.1202900